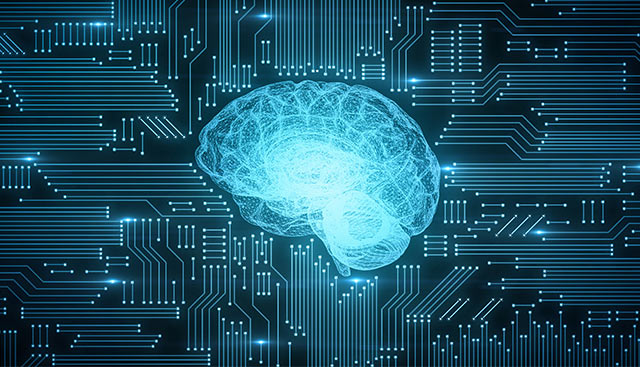
Artificial Intelligence Achieves Human-Level Performance on Several Natural Language Processing Pathology Benchmarks
In the practice of clinical oncology, most of the information in electronic medical records is unstructured text, and while natural language processing has been used to extract information from electronic medical record text, the accuracy is not optimal.
In late 2018 a powerful new deep-learning natural language processing algorithm was published: Bidirectional Encoder Representations from Transformers (BERT). BERT set new accuracy records and for the first time achieved human-level performance on several natural language processing benchmarks. At Moffitt Cancer Center, Dr. J. Ross Mitchell, Artificial Intelligence Officer, and team set a goal to train BERT to extract clinically relevant, highly accurate data from pathology reports.
Training BERT required close collaboration between a diverse, interdisciplinary team that included researchers from Moffitt’s Cancer Registry, Data Quality, Health Informatics, Biostatistics & Bioinformatics, and AI teams.
Like many cancer centers nationwide, Moffitt Cancer Center employs Certified Tumor Registrars (CTR) to collect and report data about cancer patients to state and federal agencies. The Certified Tumor Registrar-extracted data are labels that identify, with high accuracy, important information in each pathology report. Dr. Mitchell used this data to tune BERT to predetermined questions for pathology reports.
The final data-set contained 14,143 pathology reports (11,520 for training, 2,623 for testing). Moffitt’s BERT-based Q&A system searched for answers to both questions in each test report. Thus, a total of 5,246 answers were generated. Of these, 4,667 (89%) were a perfect word-for-word match with the corresponding CTR-extracted phrases. The mean F1 statistical score between the BERT answers and the CTR-extracted phrases was 92%.
Future efforts will focus on improving performance by unsupervised training of the BERT language model using 484,000 Moffitt pathology reports. The AI team will also extract additional data fields with Certified Tumor Registrar-matched ground truth labels. Ultimately new natural language processing transformer models could aid extraction of information from pathology reports and other electronic medical record documents. This, in turn, could better facilitate personalized medicine.
“A patient can come in and literally within minutes we could search their pathology reports and then we can start suggesting clinical trials they may be suitable for rather than relying on someone to go and look,” said Dr. Mitchell. “If we get the criteria for the clinical trial and we can extract this, then we are [making] a huge step towards automating matching patients to their clinical trial options.”
This study was presented at the American Association for Cancer Research Annual Meeting
To refer a patient to Moffitt, complete our online form or contact a physician liaison for assistance or support. As part of our efforts to shorten referral times as much as possible, online referrals are typically responded to within 24 - 48 hours.